Research Projects
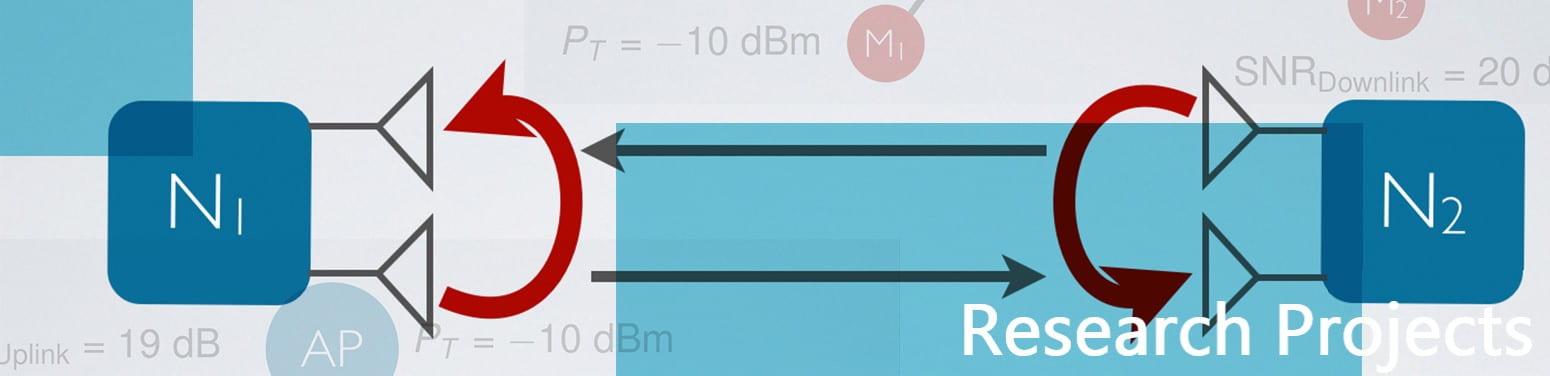
Full-Duplex Wireless
We develop foundational principles for wireless network design by leveraging full-duplex transmissions in both access and wireless backhaul. Full-duplex is most promising at shorter ranges and hence is fortuitously aligned with the predicted dominant access range in future networks. Furthermore, larger full-duplex ranges are feasible in infrastructure-to-infrastructure links and are well suited for backhaul links. While full-duplex is well-aligned with the key elements of hierarchical networks, our current design principles are largely developed for half-duplex transmissions which are the basis for all current networks.
Real-Time Machine Learning (RTML)
Recent breakthroughs in deep neural networks (DNNs) have fueled a growing demand for intelligent edge devices featuring real-time and on-site learning. However, the practical realization of such systems remains a challenge due to the limited computing and energy resources available at the edge, as well as the massive and growing learning costs for state-of-the-art DNNs.
This project’s overarching goal is to foster a systematic breakthrough in real-time machine learning (RTML) by harmonizing algorithm-, architecture-, and circuit-level innovations. The PIs will demonstrate these innovations using dronebased, on-board real-time object detection from video.
Graph Signal Processing (GSP)
Graph signal processing (GSP) is an exciting emerging field in which I have been working since my fourth year at UPenn, where I get to combine everything I learned about networks with my knowledge of classical signal processing.
Sometimes networks have intrinsic value and are themselves the object of study. In other occasions, the network defines an underlying notion of proximity, but the object of interest is a signal defined on top of the graph, i.e., data associated with the nodes of the network. This is the matter addressed in the field of graph signal processing, where the notions of, e.g., frequency and linear filtering are extended to signals supported on graphs. A plethora of graph-supported signals exist in different engineering and science fields, with examples ranging from gene expression patterns defined on top of gene networks to the spread of epidemics over a social network. Transversal to the particular application, the general goal of GSP is to contribute to the advancement of the understanding of network data by redesigning traditional tools originally conceived to study signals defined on regular domains (such as time-varying signals) and extend them to analyze signals on the more complex graph domain.
Associated Faculty
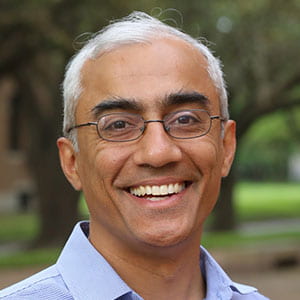
Dr. Ashutosh Sabharwal
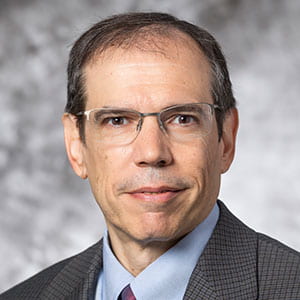
Dr. Joseph R. Cavallaro
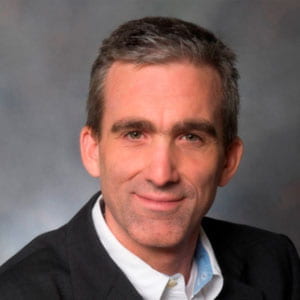
Dr. Edward Knightly
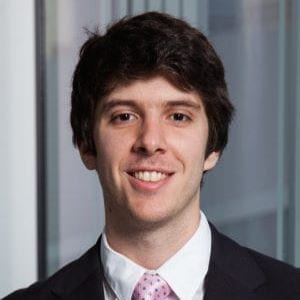
Dr. Santiago Segarra
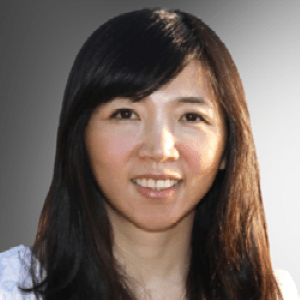
Dr. Yingyan Lin
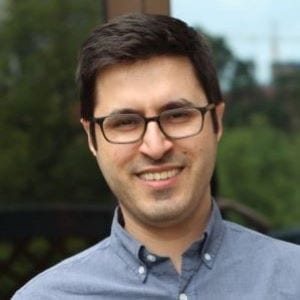